Understanding Realsense
https://github.com/IntelRealSense/librealsense/blob/master/doc/depth-from-stereo.md
Using this tutorial you will learn the basics of stereoscopic vision, including block-matching, calibration and rectification, depth from stereo using opencv, passive vs. active stereo and relation to structured light.
Why Depth?
Regular consumer web-cams offer streams of RGB data within the visible spectrum. This data can be used for object recognition and tracking, as well as some basic scene understanding.
Even with machine learning grasping the exact dimensions of physical objects is a very hard problem
This is where depth cameras come-in. The goal of a depth camera is to add a brand-new channel of information, with distance to every pixel. This new channel can be used just like the rest (for training and image processing) but also for measurement and scene reconstruction.
Stereoscopic Vision
Depth from Stereo is a classic computer vision algorithm inspired by human binocular vision system. It relies on two parallel view-ports and calculates depth by estimating disparities between matching key-points in the left and right images:
Depth from Stereo algorithm finds disparity by matching blocks in left and right images
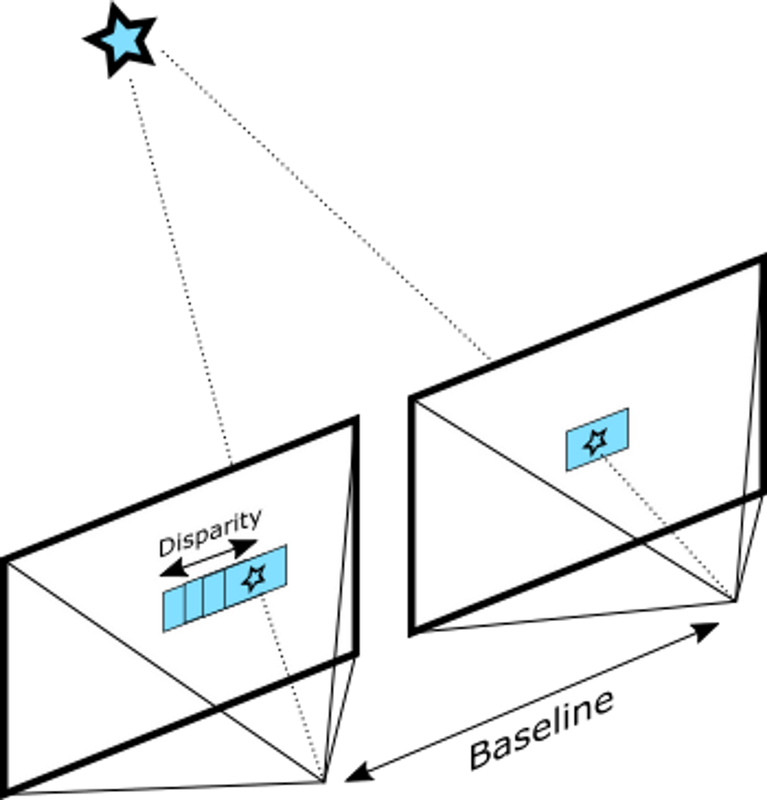
Most naive implementation of this idea is the SSD (Sum of Squared Differences) block-matching algorithm:
import numpy
fx = 942.8 # lense focal length
baseline = 54.8 # distance in mm between the two cameras
disparities = 64 # num of disparities to consider
block = 15 # block size to match
units = 0.001 # depth units
for i in xrange(block, left.shape[0] - block - 1):
for j in xrange(block + disparities, left.shape[1] - block - 1):
ssd = numpy.empty([disparities, 1])
# calc SSD at all possible disparities
l = left[(i - block):(i + block), (j - block):(j + block)]
for d in xrange(0, disparities):
r = right[(i - block):(i + block), (j - d - block):(j - d + block)]
ssd[d] = numpy.sum((l[:,:]-r[:,:])**2)
# select the best match
disparity[i, j] = numpy.argmin(ssd)
# Convert disparity to depth
depth = np.zeros(shape=left.shape).astype(float)
depth[disparity > 0] = (fx * baseline) / (units * disparity[disparity > 0])
Rectified image pair used as input to the algorithm
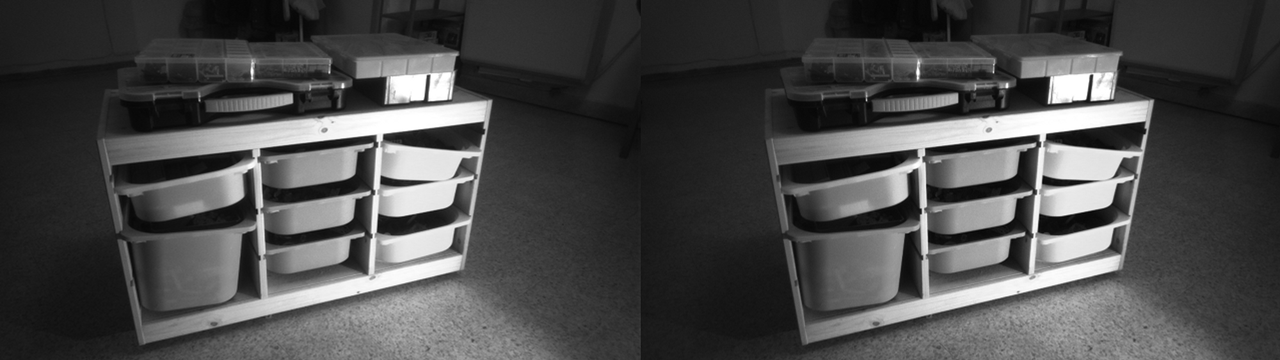
Depth map produced by the naive SSD block-matching implementation
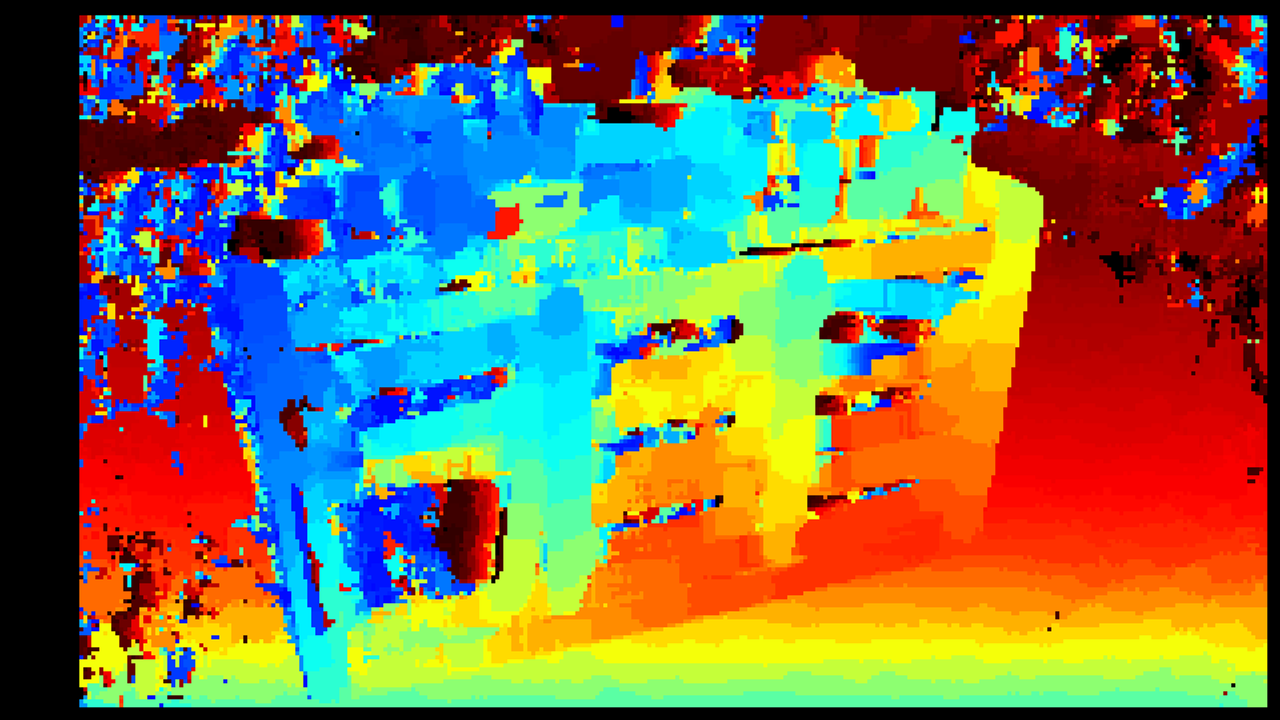
Point-cloud reconstructed using SSD block-matching
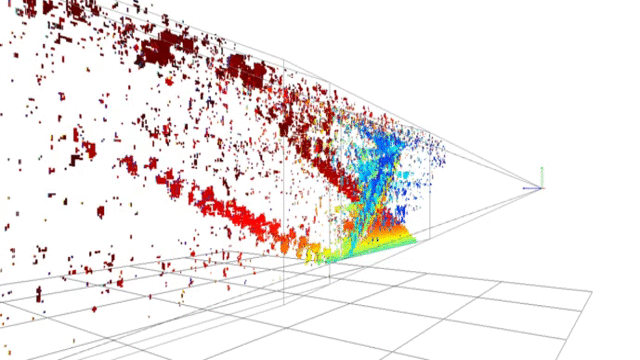
There are several challenges that any actual product has to overcome:
- Ensuring that the images are in fact coming from two parallel views
- Filtering out bad pixels where matching failed due to occlusion
- Expanding the range of generated disparities from fixed set of integers to achieve sub-pixel accuracy
Calibration and Rectification
In reality having two exactly parallel view-ports is challenging. While it is possible to generalize the algorithm to any two calibrated cameras (by matching along epipolar lines), the more common approach is image rectification. During this step left and right images are reprojected to a common virtual plane:
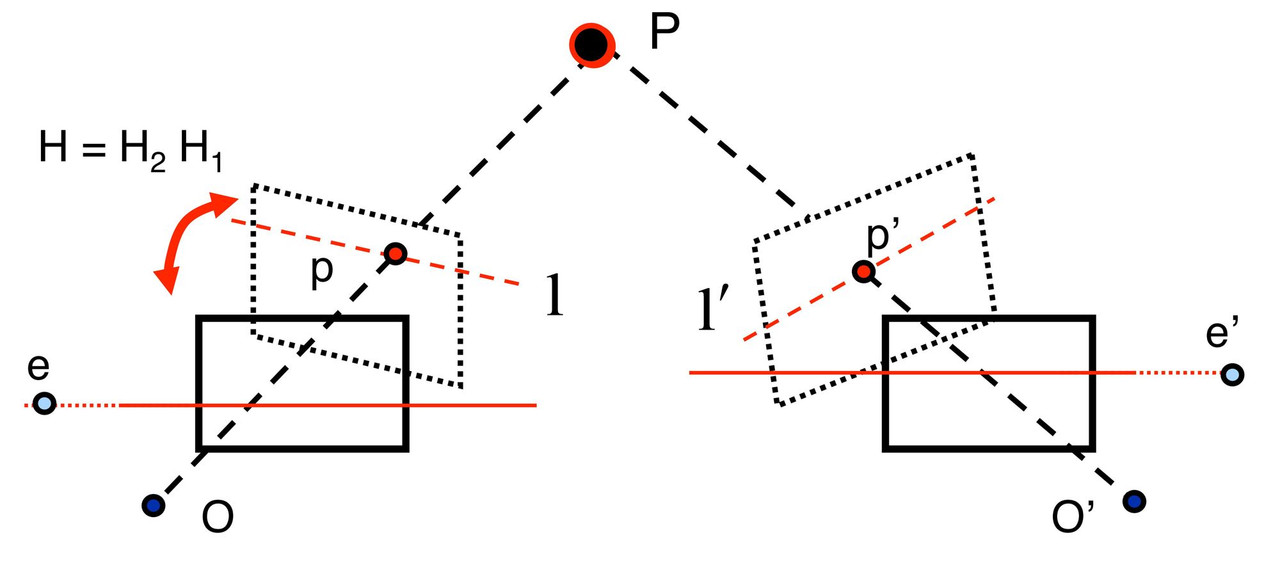
Software Stereo
opencv library has everything you need to get started with depth:
- calibrateCamera can be used to generate extrinsic calibration between any two arbitrary view-ports
- stereorectify will help you rectify the two images prior to depth generation
- stereobm(number of disparity clarification) and stereosgbm(Stereo processing by semiglobal matching and mutual information
) can be used for disparity calculation
- reprojectimageto3d to project disparity image to 3D space
import numpy
import cv2
fx = 942.8 # lense focal length
baseline = 54.8 # distance in mm between the two cameras
disparities = 128 # num of disparities to consider
block = 31 # block size to match
units = 0.001 # depth units
sbm = cv2.StereoBM_create(numDisparities=disparities,
blockSize=block)
disparity = sbm.compute(left, right)
depth = np.zeros(shape=left.shape).astype(float)
depth[disparity > 0] = (fx * baseline) / (units * disparity[disparity > 0])
Passive vs Active Stereo
The quality of the results you will get with this algorithm depends primarily on the density of visually distinguishable points (features) for the algorithm to match. Any source of texture, natural or artificial will improve the accuracy significantly.
That’s why it is extremely useful to have an optional texture projector (usually adding details outside of the visible spectrum). As an added benefit, such projector can be used as an artificial source of light at night or in the dark.
Input images illuminated with texture projector
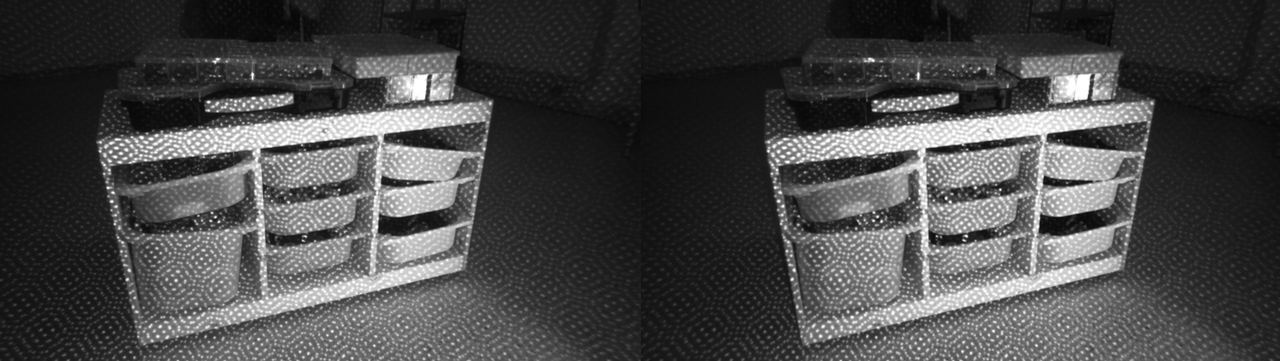
Left: opencv stereobm without projector. Right: stereobm with projector.
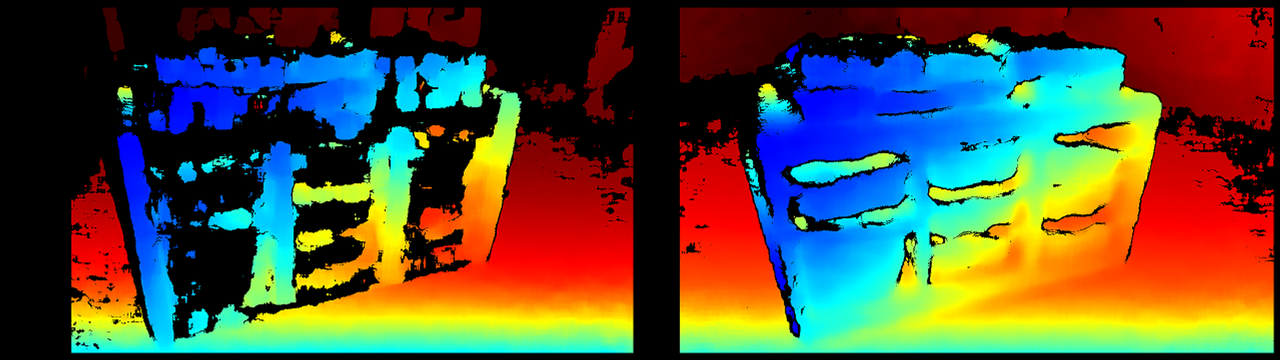
Structured-Light is an alternative approach to depth from stereo. It relies on recognizing a specific projected pattern in a single image.
For customers interested in a structured-light solution Intel offers the SR300 RealSense camera
Having certain benefits, structured-light solutions are known to be fragile since any external interference (from either sunlight or another structured-light device) will prevent you from getting any depth.
In addition, since laser projector has to illuminate the entire scene, power consumption grows with range, often requiring a dedicated power source.
Depth from stereo on the other hand, only benefits from multi-camera setup and can be used with or without projector.
D400 Intel RealSense cameras
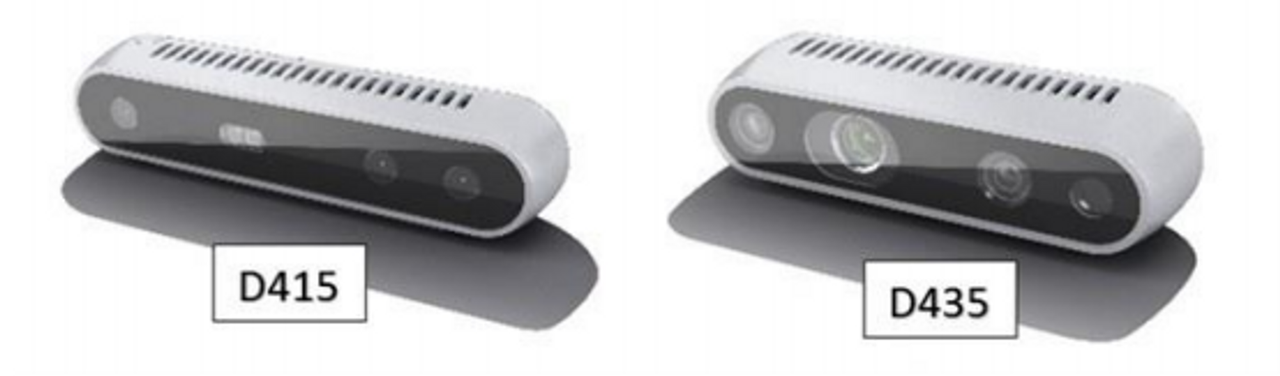
D400 RealSense cameras offer the following basic features:
- The device comes fully calibrated producing hardware-rectified pairs of images
- All depth calculations is done by the camera at up-to 90 FPS
- The device offers sub-pixel accuracy and high fill-rate
- There is an on-board texture projector for tough lighting conditions
- The device runs of standard USB 5V power-source drawing around 1-1.5 W
This product was designed from the ground up to address conditions critical to robotics / drones developers and to overcome the limitations of structured light.
Depth-map using D415 Intel RealSense camera
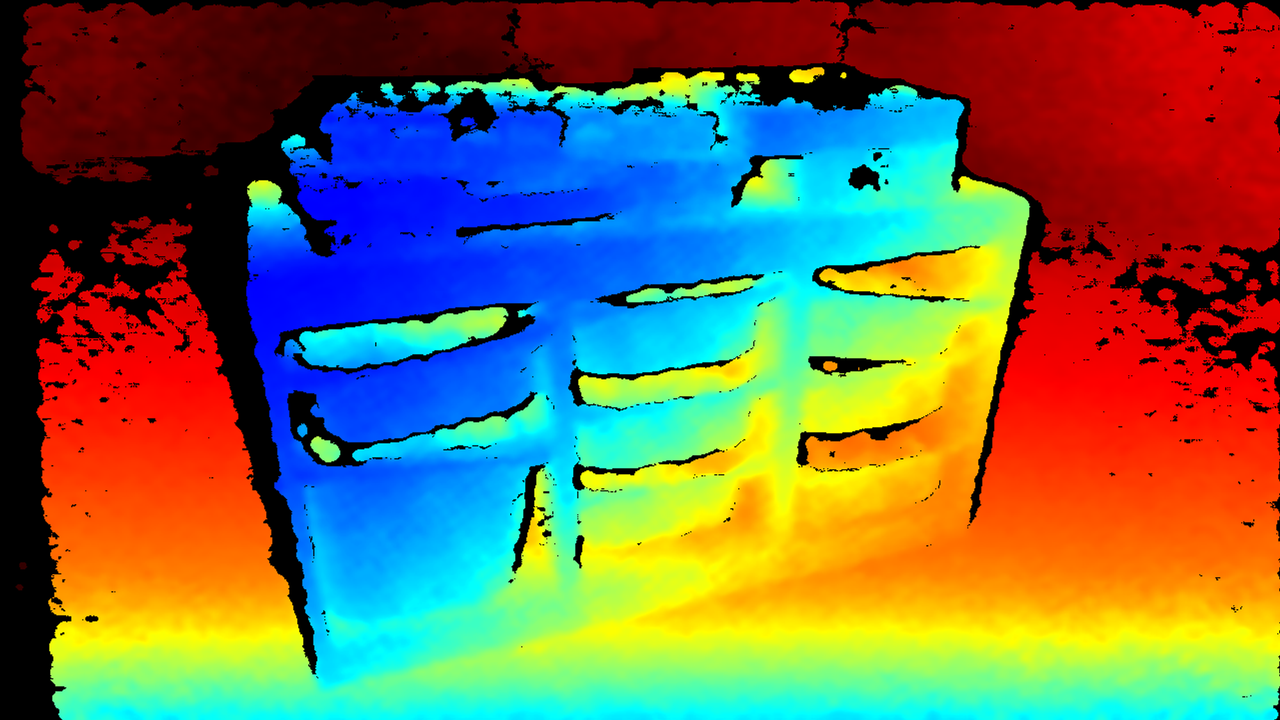
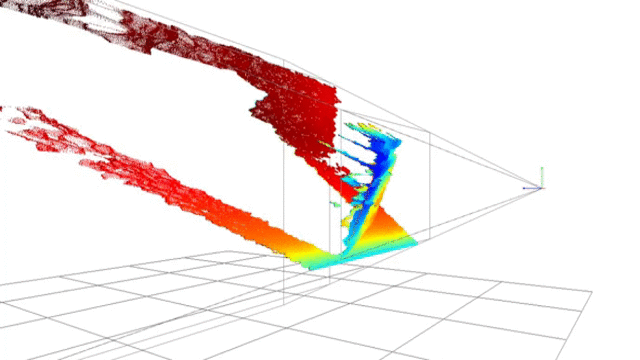
realsense pattern matching
because of realsesne depth image data generated by pattern matching between stereo camera and structed light way, if camera seeing some pattern scene, dept data will be generated in wrong because of pattern matching way.
Realsense Docs
https://github.com/IntelRealSense/librealsense/tree/master/doc
What is active infrared (IR) stereo
The camera “sees” both IR and visible light and performs dense stereo matching based on features it can see. In visible wavelength, such features would be visible features, like corners, texture, edges, etc…
In infrared, it also can pick up on artificial features generated by the projector (in addition to natural features)
https://www.researchgate.net/figure/The-depth-perception-based-on-active-infrared-stereo-vision-technology_fig2_328507744
To improve the 3D point cloud modeling of complex indoor environment, a novel point cloud
registration method based on IntelR RealSenseTM depth camera is proposed in this paper, which
can reduce the influence of measuring errors. The IntelR RealSenseTM depth camera adopts active
infrared (IR) stereo vision technology, which is shown in Figure 2. The depth perception based on
stereo vision is implemented by two image sensors and an infrared projector. The infrared projector
projects non-visible structured IR pattern to improve depth accuracy in scenes. The depth calculation
process in presented in the right of Figure 2. The depth image processor obtains the scene data by the
two image sensors, and the depth values for each pixel can be calculated by correlating the points on
the left image to the right image.
https://www.google.com/imgres?imgurl=https%3A%2F%2Fwww.laser2000.de%2F518-thickbox_default%2Fosela-random-pattern-projector-the-premium-laser-for-structured-lighting.jpg&imgrefurl=https%3A%2F%2Fwww.laser2000.de%2Fen%2Flaser-diode-modules%2F344-osela-random-pattern-projector-the-premium-laser-for-structured-lighting.html&tbnid=KBpXOuVs7WZ2KM&vet=12ahUKEwi_i7ScqMT2AhWRTPUHHbxmBFsQMygLegUIARDOAQ..i&docid=A9kYlC-CWSts5M&w=800&h=800&q=structured IR pattern&hl=en&ved=2ahUKEwi_i7ScqMT2AhWRTPUHHbxmBFsQMygLegUIARDOAQ
[https://www.google.com/imgres?imgurl=https%3A%2F%2Fstatic-01.hindawi.com%2Farticles%2Fjs%2Fvolume-2014%2F852621%2Ffigures%2F852621.fig.001.jpg&imgrefurl=https%3A%2F%2Fwww.hindawi.com%2Fjournals%2Fjs%2F2014%2F852621%2F&tbnid=6JxHaQD2t-4Z7M&vet=12ahUKEwi_i7ScqMT2AhWRTPUHHbxmBFsQMygCegUIARC8AQ..i&docid=8-jTry2NDHZNM&w=600&h=360&q=structured IR pattern&hl=en&ved=2ahUKEwi_i7ScqMT2AhWRTPUHHbxmBFsQMygCegUIARC8AQ](https://www.google.com/imgres?imgurl=https%3A%2F%2Fstatic-01.hindawi.com%2Farticles%2Fjs%2Fvolume-2014%2F852621%2Ffigures%2F852621.fig.001.jpg&imgrefurl=https%3A%2F%2Fwww.hindawi.com%2Fjournals%2Fjs%2F2014%2F852621%2F&tbnid=6JxHaQD2t-4Z7M&vet=12ahUKEwi_i7ScqMT2AhWRTPUHHbxmBFsQMygCegUIARC8AQ..i&docid=8-jTry2NDHZNM&w=600&h=360&q=structured%20IR%20pattern&hl=en&ved=2ahUKEwi_i7ScqMT2AhWRTPUHHbxmBFsQMygCegUIARC8AQ)